Introduction
Understanding customer behavior is critical for businesses aiming to improve engagement, enhance customer satisfaction, and drive revenue. Traditional customer analysis methods are no longer sufficient in today’s fast-paced digital landscape. Companies now rely on predictive analytics in CRM to anticipate customer needs, personalize interactions, and optimize business strategies.
CRM predictive modeling uses historical and real-time data to forecast customer behaviors, enabling businesses to proactively address issues, maximize sales opportunities, and strengthen customer relationships. In this article, we will explore how predictive analytics can anticipate customer needs, the benefits of CRM data analytics, and best practices for leveraging CRM for customer behavior forecasting.
What is Predictive Analytics in CRM?
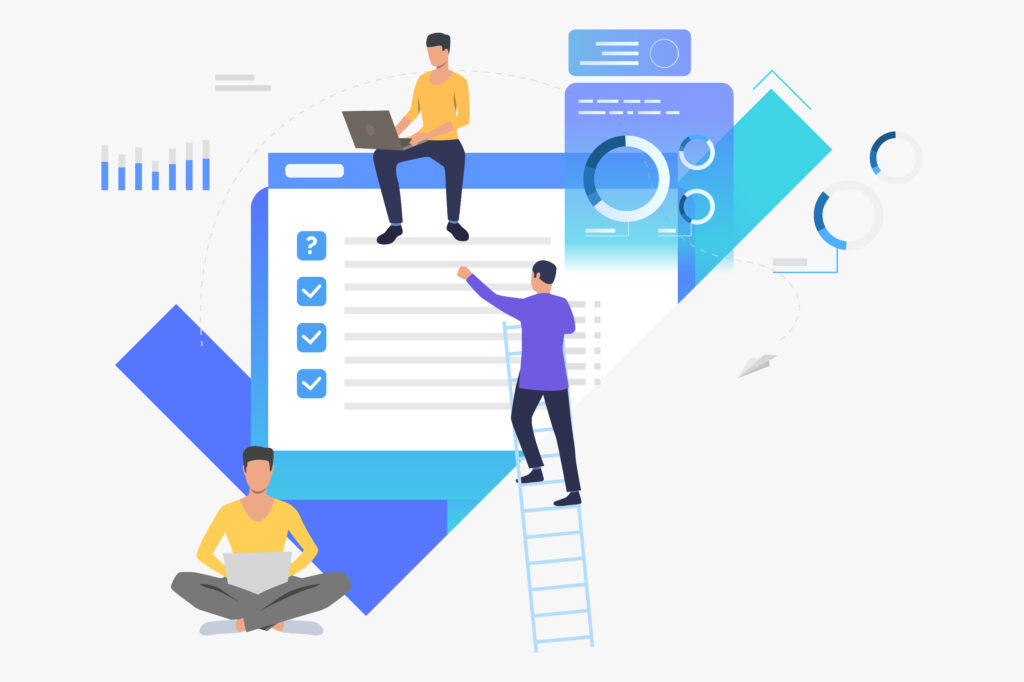
Predictive analytics in CRM involves the use of artificial intelligence (AI), machine learning, and statistical algorithms to analyze past customer data and predict future behaviors. By examining purchase history, browsing patterns, and interaction data, businesses can:
- Identify potential leads and high-value customers
- Forecast customer churn and take preventive actions
- Personalize marketing campaigns based on behavior predictions
This data-driven approach transforms CRM systems from simple record-keeping tools into powerful engines for customer behavior prediction and strategic decision-making.
How Predictive Analytics Can Anticipate Customer Needs
Predictive analytics enables businesses to stay ahead of customer expectations by identifying patterns and trends that indicate future actions. Here’s how businesses can use CRM predictive modeling to anticipate customer needs:
1. Customer Segmentation for Targeted Marketing
By analyzing customer data, predictive analytics segments customers into distinct groups based on purchasing behavior, demographics, and engagement levels. This allows businesses to:
- Develop hyper-targeted marketing campaigns
- Deliver personalized product recommendations
- Optimize pricing strategies for different customer segments
2. Forecasting Customer Churn
Retaining existing customers is more cost-effective than acquiring new ones. Predictive analytics helps identify at-risk customers by analyzing:
- Declining engagement levels
- Reduced purchase frequency
- Negative sentiment in customer feedback
Once a potential churn risk is detected, businesses can take proactive measures, such as offering personalized discounts, enhancing customer support, or re-engaging through tailored email campaigns.
3. Product Demand Prediction
CRM predictive modeling helps businesses forecast demand for specific products or services based on:
- Seasonal trends
- Past purchase history
- Emerging market behaviors
Retailers, for example, can stock inventory more efficiently, ensuring they meet demand without overstocking or understocking.
4. Enhancing Customer Support with AI
Predictive analytics improves customer service by analyzing previous interactions and predicting potential issues. AI-powered CRM solutions can:
- Recommend solutions based on similar past queries
- Automatically escalate complex cases to human agents
- Provide proactive support, such as reminding customers of upcoming renewals or potential issues with their subscriptions
5. Optimizing Sales Strategies
Sales teams can use predictive analytics in CRM to:
- Identify high-value leads
- Determine the best time and method to reach out to prospects
- Personalize sales pitches based on customer preferences and behaviors
With these insights, sales representatives can close deals more efficiently and increase conversion rates.
Using CRM for Customer Behavior Forecasting
Customer behavior forecasting leverages CRM data analytics to predict future actions, enabling businesses to create more strategic marketing and sales plans. Some key techniques include:
1. Machine Learning for Behavior Prediction
Machine learning algorithms analyze large datasets to identify patterns and predict future actions. Businesses can apply machine learning models to:
- Detect anomalies in customer behavior
- Predict likelihood of purchase
- Recommend personalized content
2. Sentiment Analysis for Customer Insights
CRM systems can analyze customer reviews, social media interactions, and survey responses to gauge customer sentiment. This helps businesses:
- Identify pain points and address issues proactively
- Improve product offerings based on customer feedback
- Strengthen brand reputation by responding to concerns in real-time
3. Next-Best-Action Recommendations
CRM predictive modeling suggests the best course of action for engaging customers based on past interactions. This could include:
- Sending a follow-up email to a lead showing high engagement
- Offering a discount to a customer who abandoned their shopping cart
- Providing upsell or cross-sell suggestions during customer interactions
4. Personalized Customer Journeys
CRM analytics create personalized customer journeys by analyzing behavioral data. Businesses can:
- Automate tailored email sequences
- Adjust content recommendations based on browsing habits
- Deliver targeted ads that align with customer interests
5. Dynamic Pricing Strategies
Predictive analytics helps businesses optimize pricing by analyzing market trends, competitor pricing, and customer willingness to pay. This allows for:
- Real-time pricing adjustments
- Personalized discounts based on customer loyalty
- Demand-based price optimization
Benefits of CRM Data Analytics in Understanding Customer Behavior
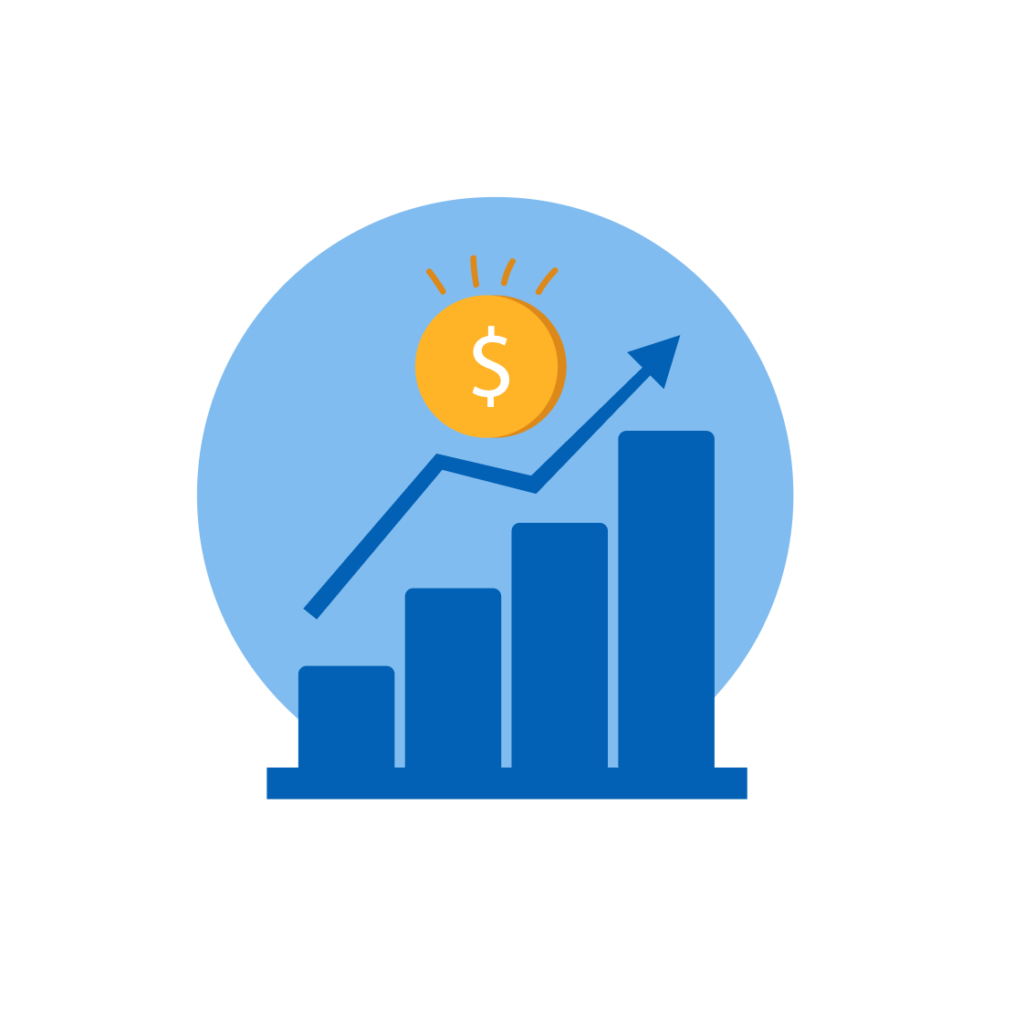
Integrating predictive analytics with CRM systems provides businesses with numerous advantages:
1. Improved Customer Experience
By anticipating customer needs, businesses can provide timely and relevant interactions, enhancing overall customer satisfaction.
2. Increased Sales and Revenue
Predictive analytics helps businesses focus on high-potential leads, personalize sales pitches, and optimize marketing campaigns, resulting in higher conversion rates.
3. Better Resource Allocation
Businesses can allocate resources more effectively by prioritizing high-value customers, reducing wasteful marketing spend, and optimizing inventory management.
4. Enhanced Customer Retention
Proactively addressing customer concerns and providing personalized offers helps improve retention and loyalty.
5. Competitive Advantage
Companies that leverage CRM predictive modeling gain a strategic edge by making data-driven decisions that align with customer expectations.
Challenges in Implementing Predictive Analytics in CRM
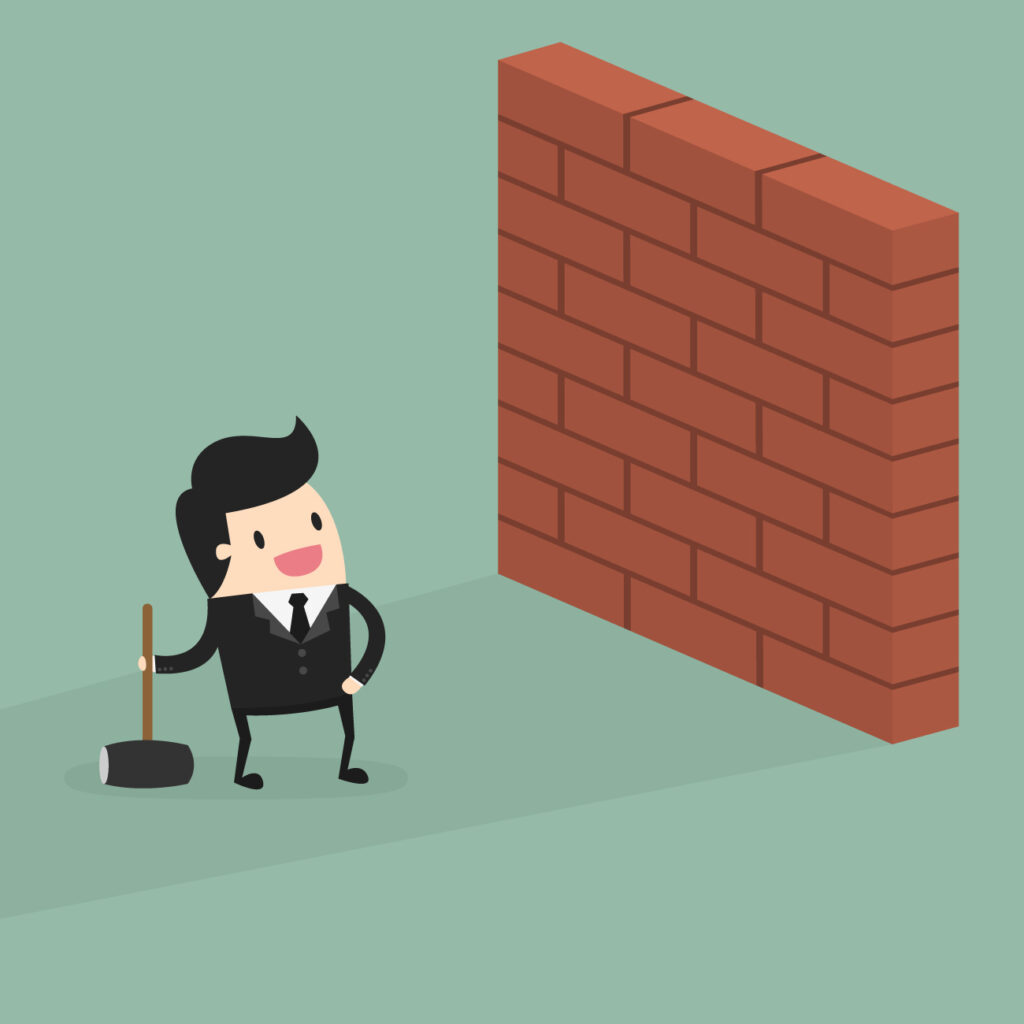
Despite its benefits, integrating predictive analytics in CRM comes with challenges. Here’s how to overcome them:
1. Data Quality Issues
Incomplete or inaccurate data can impact predictions. Businesses should:
- Ensure data hygiene by regularly cleaning and updating CRM records
- Use data validation techniques to minimize errors
2. Integration Complexity
Many businesses struggle to integrate predictive analytics tools with their existing CRM. Solutions include:
- Choosing CRM platforms with built-in analytics capabilities
- Working with CRM vendors or consultants for smooth integration
3. Privacy and Security Concerns
Predictive analytics requires large volumes of customer data, raising privacy concerns. Businesses should:
- Comply with data protection regulations (e.g., GDPR, CCPA)
- Implement strong encryption and access control measures
4. Resistance to Change
Employees may resist adopting AI-driven CRM analytics due to fear of complexity or job displacement. To address this:
- Provide training on the benefits of predictive analytics
- Position AI as a support tool rather than a replacement
The Future of Predictive Analytics in CRM
As AI and machine learning continue to advance, predictive analytics in CRM will become even more sophisticated. Future trends include:
1. AI-Powered Chatbots for Proactive Engagement
AI-driven chatbots will analyze customer data to initiate personalized conversations and resolve issues before they escalate.
2. Real-Time Predictive Insights
Businesses will move towards real-time analytics, enabling instant decision-making based on live customer interactions.
3. Voice and Emotion Recognition
Advanced AI tools will analyze customer voice tones and emotions to assess sentiment and personalize interactions accordingly.
4. Automated Hyper-Personalization
AI-driven automation will deliver ultra-personalized experiences based on real-time behavior tracking.
Conclusion
Predictive analytics in CRM is transforming the way businesses anticipate customer needs and improve engagement. By leveraging CRM predictive modeling, companies can forecast customer behavior, optimize marketing efforts, and enhance sales strategies. As technology advances, businesses that adopt CRM data analytics will gain a competitive edge in understanding and serving their customers better. Now is the time to embrace predictive analytics and unlock the full potential of your CRM system.
